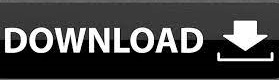
Structure-based machine learning methods for design thus far have focused on mutation prediction 25, 26, 27, 28, 29, 30, rotamer repacking of native sequences 31, or amino acid sequence design without modeling side-chain conformers 32, 33, 34, 35, with some experimental validation including circular dichroism data 33 and fluorescence 26. We hypothesized that by training a model that conditions on local backbone structure and chemical environment, the network might learn residue-level patterns that allow it to generalize without fine-tuning to new backbones with topologies outside of the training distribution, opening up the possibility for generation of de novo designed sequences with novel structures and functions. Recent experimentally validated efforts for machine learning-based sequence generation have focused on sequence representation learning without structural information, requiring fitting to data from experiments or from known protein families to produce functional designs 23, 24. With the emergence of deep learning systems and their ability to learn patterns from high-dimensional data, it is now possible to build models that learn complex functions of protein sequence and structure, including models for protein backbone generation 18, 19, 20 and protein structure prediction 21, 22 as a result, we were curious as to whether an entirely learned method could be used to design protein sequences on par with energy function methods. Current approaches for fixed-backbone design commonly involve specifying an energy function and sampling sequence space to find a minimum-energy configuration 13, 14, 15, and enormous effort has gone into the development of carefully modeled and parameterized energy functions to guide design, which continue to be iteratively refined 16, 17. The functional design of enzymes, ligand binding sites, and interfaces all require fine-grained control over side-chain types and conformations. This difficult task 12 is often described as the inverse of protein folding-given a protein backbone, design a sequence that folds into that conformation. Key to such successes is robust sequence design methods that minimize the folded-state energy of a pre-specified backbone conformation, which can either be derived from existing structures or generated de novo. Computational protein design has emerged as a powerful tool for rational protein design, enabling significant achievements in the engineering of therapeutics 1, 2, 3, biosensors 4, 5, 6, enzymes 7, 8, and more 9, 10, 11.
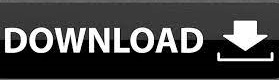